キーエンスの
データ活用ノウハウ
を凝縮したソフトウェア
データアナリティクス プラットフォーム KIシリーズ
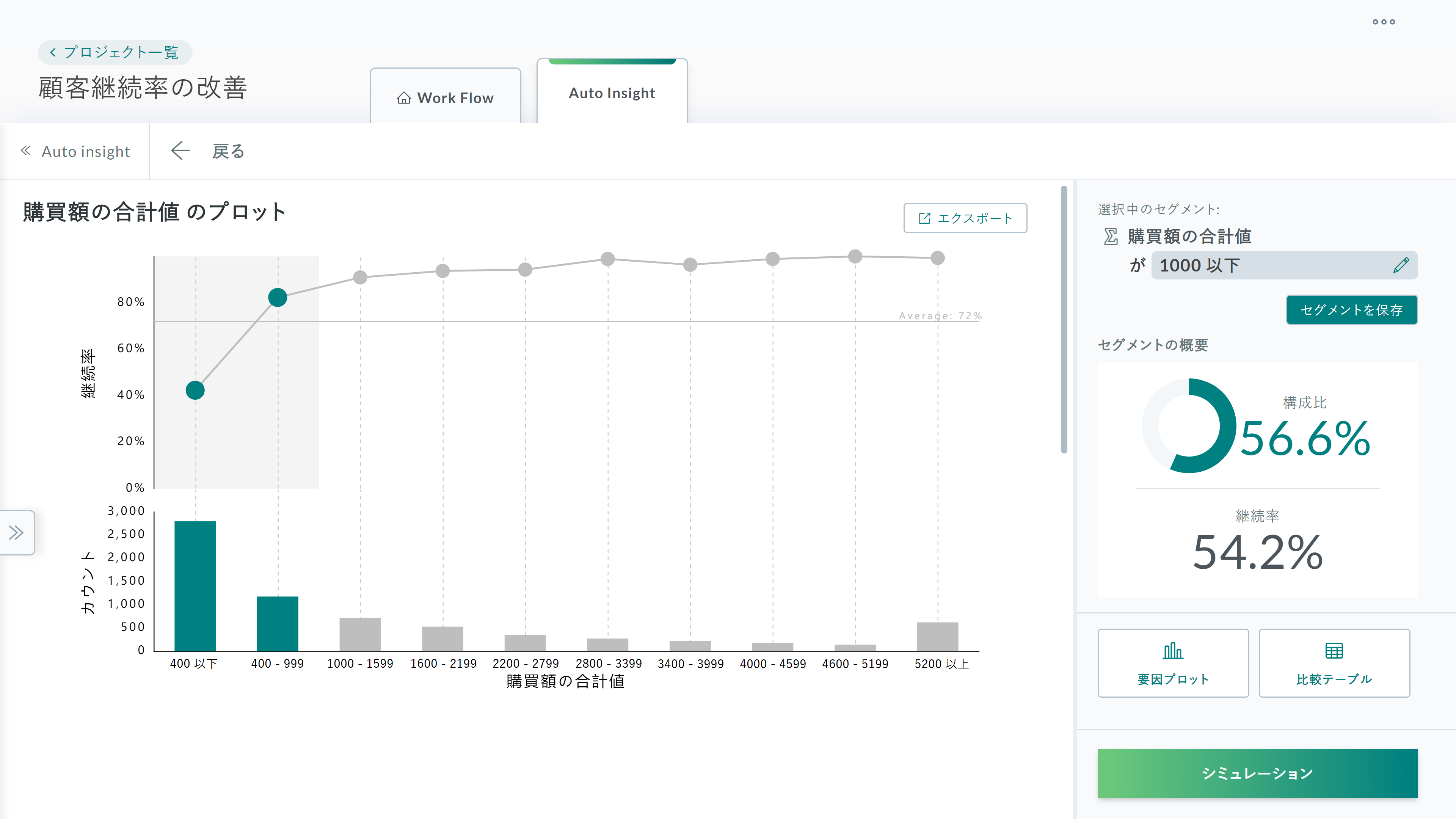
誠に勝手ながら、以下の期間を休業とさせていただきます。
2024年4月27日(土)~2024年5月6日(月)
休業中にいただいた「お問い合わせ」につきましては、
5月7日(火)以降に対応させていただきます。
また、4月26日(金)にいただいた「お問い合わせ」につきましても、
5月7日(火)以降に対応させていただく場合がございます。
大変ご迷惑をおかけしますが、何卒ご了承くださいますようお願い申し上げます。
導入事例/お客様の声
セミナー/イベント
-
お申し込み受付中 ウェビナー
[05月14日(火) 10:00] はじめてのKI(30分版)
-
お申し込み受付中 ウェビナー
[05月14日(火) 11:10] ③キーエンス流「データ活用7つのヒント」(生放送)
-
お申し込み受付中 ウェビナー
[05月14日(火) 13:15] “今あるデータ”から始めよう!【ちょビナー・データサイエンティストに聞く】
膨大なデータのなかから、
ビジネス課題を
解決するための
有効な
”打ち手”を見つけ出す
KIシリーズはデータを入れて解決したいビジネス課題を入力するだけで施策が生まれる、
ビジネスユーザ向けのデータ分析ソフトウェアです。
膨大なデータの中から有効な切り口を自動で見つけることで、
的確かつスピーディーに最も効果的な施策を打つことができるようになります。
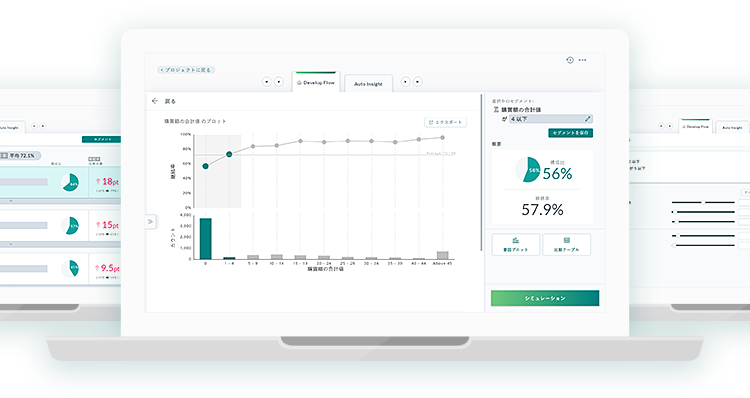
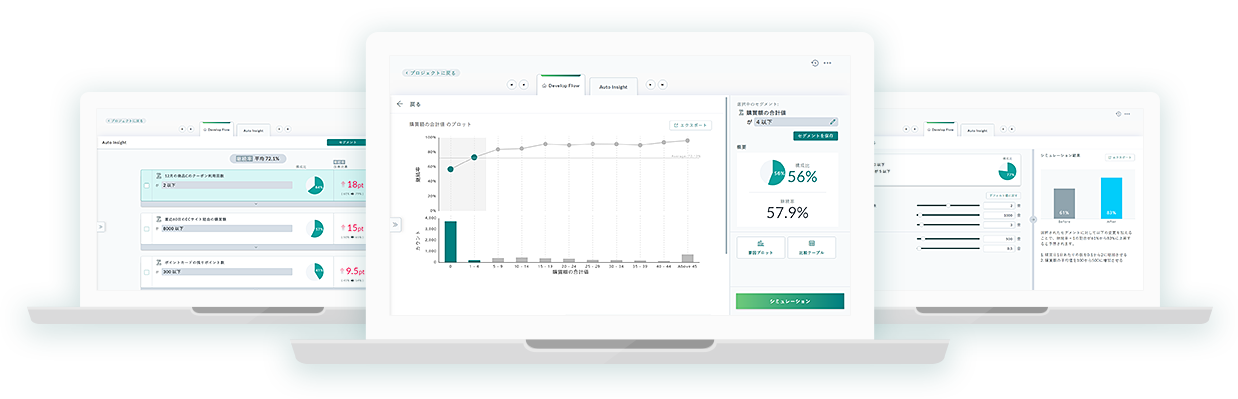
データ分析が変われば
施策も変わる
ビジネス課題:
「リピート顧客を増やしたい」の場合…
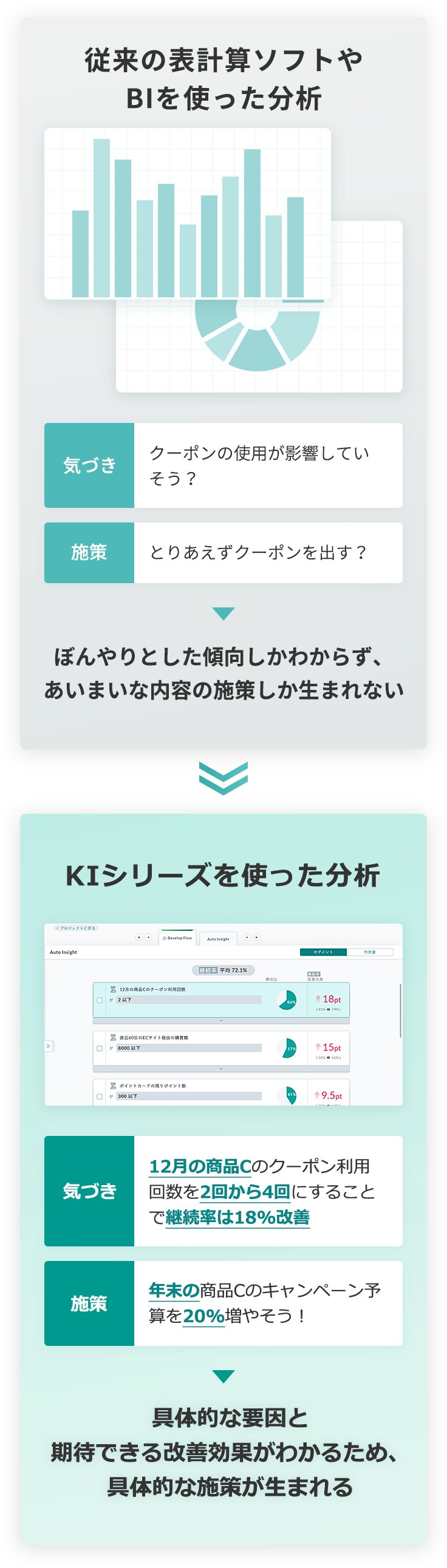
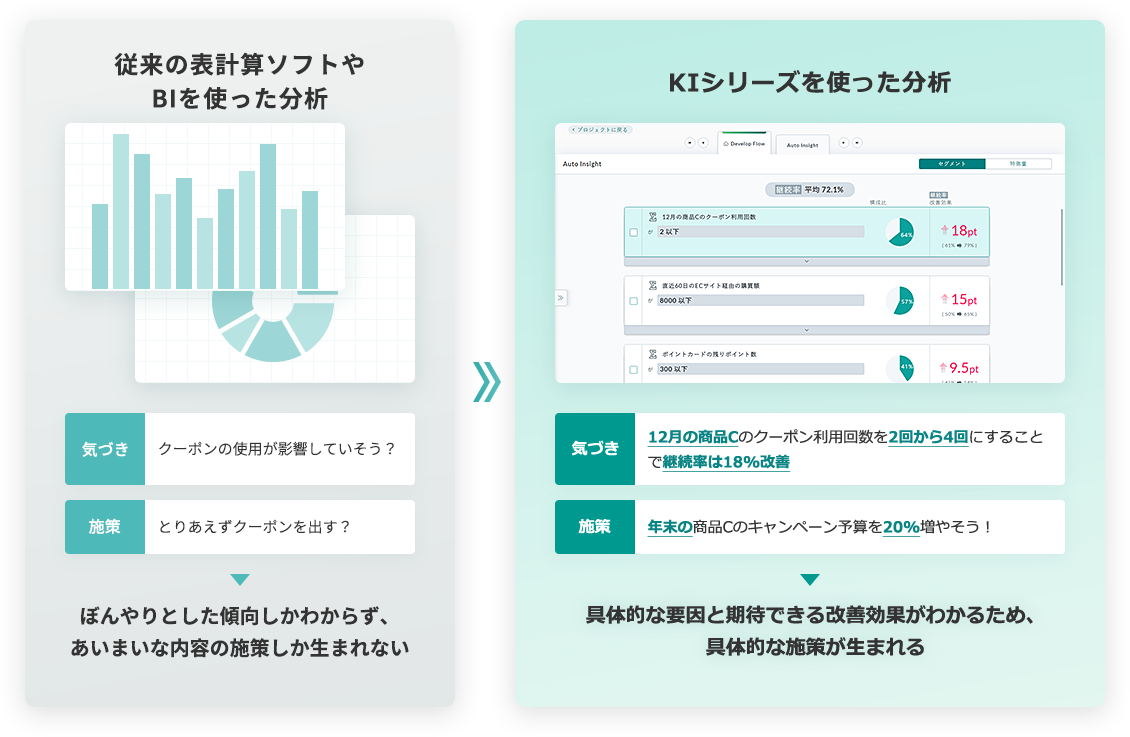
データを入れるだけ。
無数の施策切り口を自動的に作り出します
-
データを使える形に
プログラムなしでバラバラなデータを活用できるカタチへ変換します。
-
施策の切り口を
自動生成人では思いつくことが困難な無数の切り口を自動生成します。
-
施策の切り口を
ランキング切り口を機械学習で順位づけ。重要な切り口が見つかります。
-
施策シミュレーション
施策の改善効果をシミュレーション。効果の大きさが事前にわかります。
あなたのビジネス課題を
解決します
-
マーケティングの
パーソナライゼーションお客様の行動や嗜好から最適なオファーを実施し、マーケティング施策のROIを高めます。
-
クーポン・値引き施策
お客様、商品ごとに最適なクーポン提示や値引きを行い、利益最大化を図ります。
-
顧客の離反防止・長期顧客化
離反に至りやすいお客様の属性や行動パターンを理解し、長期顧客化への打ち手を見つけます。
-
営業活動の効率化
営業活動データ・顧客データと購買データから成約パターンを分析し、確率の高い営業活動を行います。
-
商品展開プランの最適化
お客様の行動やライフスタイルに共通する特徴を見つけ、ターゲットに即した商品展開プランを作成できます。
-
不良発生要因の分析
生産条件や材料などの不良発生の要因を分析することで、品質改善への根本対策を講じることができます。
キーエンスについて

キーエンスはモノづくりをサポートするファクトリーオートメーション業界のグローバルリーディングカンパニーです。
1974年の会社設立以来、「付加価値の創造」によって社会へ貢献するという考えのもと事業を展開してきました。キーエンスが大切にしていることとして、社名の由来である「Key of Science」にも表れるように、企業活動をデータで科学的に捉え、合理的な判断をしよう、という考えがあります。
KIシリーズはキーエンスにおける「データ活用のノウハウ」を具現化した、ビジネスユーザ向けの実践的データ分析ソフトウェアです。
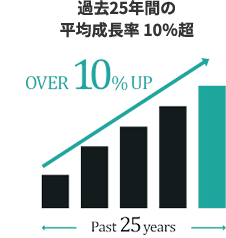